Learn to Code in R: Reading in External Data Files
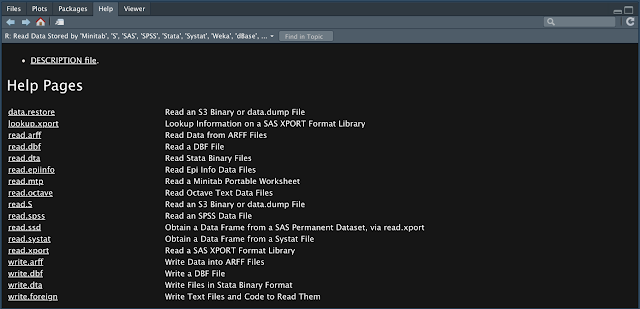
One skill that everyone in R should have is how to read in external data files. Many people who have some exposure to R will have some familiarity with this skill, but little knowledge of the many formats R can handle. This is often because many people's exposure is from a singular class or a project they did once. My hope is to provide the reader with a broader understanding of R's ability to handle a number of data formats. In this post, I will cover, How to read in .csv, Stata, SPSS, SAS, and Excel spreadsheet files. Some formatting options and different abilities you ought to know. Some explanations regarding help documentation and using function arguments/options. Saving and loading Rdata files for minimal hassle once the data is just the way you want it. Reading in Text Files and Function Options The basic function for reading in data is read.table() . I mention this one first because the other functions for reading in external data are based off of this one. In ...